-
Notifications
You must be signed in to change notification settings - Fork 4
Commit
This commit does not belong to any branch on this repository, and may belong to a fork outside of the repository.
Showing
15 changed files
with
703 additions
and
17 deletions.
There are no files selected for viewing
This file contains bidirectional Unicode text that may be interpreted or compiled differently than what appears below. To review, open the file in an editor that reveals hidden Unicode characters.
Learn more about bidirectional Unicode characters
File renamed without changes
Loading
Sorry, something went wrong. Reload?
Sorry, we cannot display this file.
Sorry, this file is invalid so it cannot be displayed.
File renamed without changes
File renamed without changes
This file contains bidirectional Unicode text that may be interpreted or compiled differently than what appears below. To review, open the file in an editor that reveals hidden Unicode characters.
Learn more about bidirectional Unicode characters
This file contains bidirectional Unicode text that may be interpreted or compiled differently than what appears below. To review, open the file in an editor that reveals hidden Unicode characters.
Learn more about bidirectional Unicode characters
This file contains bidirectional Unicode text that may be interpreted or compiled differently than what appears below. To review, open the file in an editor that reveals hidden Unicode characters.
Learn more about bidirectional Unicode characters
This file contains bidirectional Unicode text that may be interpreted or compiled differently than what appears below. To review, open the file in an editor that reveals hidden Unicode characters.
Learn more about bidirectional Unicode characters
This file contains bidirectional Unicode text that may be interpreted or compiled differently than what appears below. To review, open the file in an editor that reveals hidden Unicode characters.
Learn more about bidirectional Unicode characters
Original file line number | Diff line number | Diff line change |
---|---|---|
@@ -0,0 +1,41 @@ | ||
# Parameter-Efficient Finetuning for LLMs | ||
|
||
## 1. Low Ranking Adaptation (LoRA) | ||
|
||
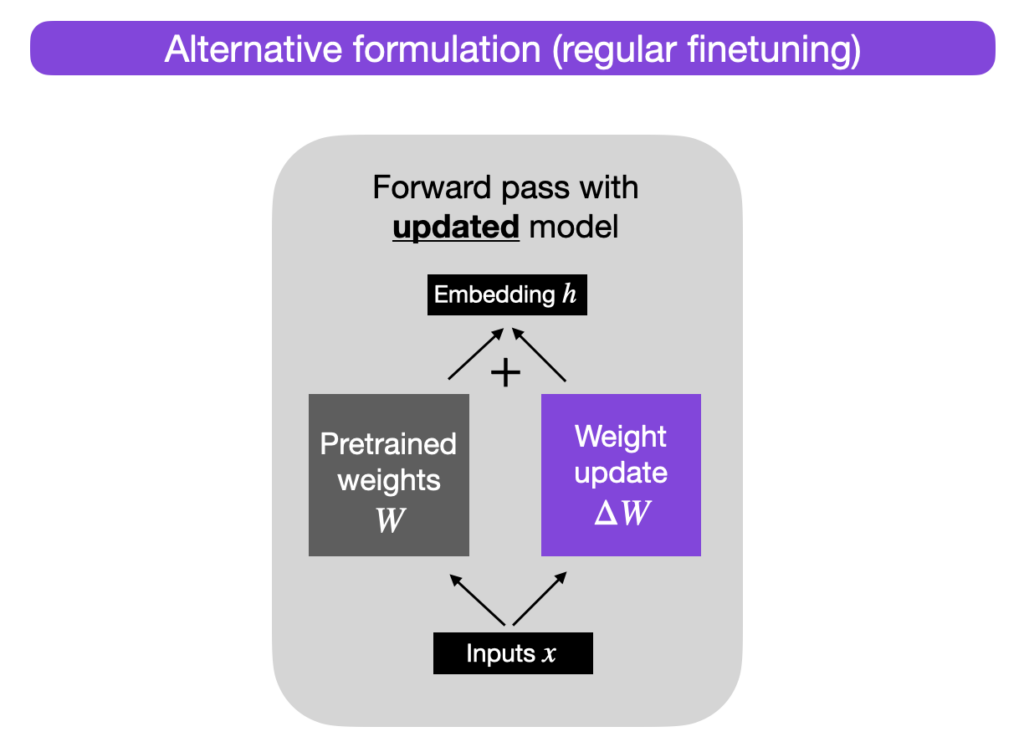 | ||
pic 1: LoRA architecture[^1] | ||
|
||
Low ranking adaptation is a technique for parameter-efficient finetuning of large language models. Instead of updating all the weights during finetuning (which is very compute intensive) we can learn a separate matrix that will store updates of pretrained weights and in order to reduce number of computations we as well decompose this weight update matrix into two matrices of a lower rank. | ||
|
||
As an example: we can have pretrained weights of shape (d, d) and two matrices A and B of shape (d, r) and (r, d) respectively; pretreined weights will not be changed during training, only matrices A and B. | ||
As it can be seen in the scheme above we apply pretrained weights on input, also apply separate weight update matrix formed by A@B matrix multiplication and then do summation. | ||
|
||
If d=100 and r=1, then instead of updating 100x100=10_000 parameters (for pretrained weights) we will update only 100x1 (for matrix A) and 1x100 (for matrix B) which makes it in total 200 paremeters to update instead of 10k. | ||
|
||
More about it you can read in: | ||
|
||
1. Microsoft's LoRA | ||
- [paper](https://arxiv.org/pdf/2106.09685.pdf) | ||
- [repo](https://github.com/microsoft/LoRA/) | ||
2. Lighting.ai | ||
- [blogpost](https://lightning.ai/pages/community/tutorial/lora-llm/) | ||
- [repo](https://github.com/Lightning-AI/lit-llama) | ||
|
||
### -- About implementation -- | ||
|
||
If one take a look at the Microsoft's LoRA repo then can notice that there are two classes: `Linear` and `MergedLinear` and it can be confusing in the beginning. | ||
|
||
Basically there are two approaches of calculating query, key and value matrices: | ||
|
||
In the basic implementation of attention mechanism you have three separate weight matrices for query, key and | ||
value and thus in order to obtain q, k, v you apply these 3 matrices separately on input x (in self-attention). | ||
This approach is covered with LoRA.Linear class. It's not implemented in this repository, but one can find implementation [here](https://github.com/microsoft/LoRA/blob/main/loralib/layers.py#L91). | ||
|
||
The other approach is to have a single matrix that stores weights for all three matrices (query, key and value). | ||
So you can apply this big combined matrix ones (helps with parallelization on GPU) and then you can split the | ||
output into three chunks to have queries, keys and values. To cover this approach MergedLinear class is created. | ||
|
||
Note: examples of calculating qkv matrices with separate multiplications and a single one you can find in | ||
`attention.py` files of this repository. | ||
|
||
[^1]: [Parameter-Efficient LLM Finetuning With Low-Rank Adaptation (LoRA) by Lightning.ai](https://lightning.ai/pages/community/tutorial/lora-llm/) |
Oops, something went wrong.