You signed in with another tab or window. Reload to refresh your session.You signed out in another tab or window. Reload to refresh your session.You switched accounts on another tab or window. Reload to refresh your session.Dismiss alert
I want to know how to make baseline model of MCUNetV2 paper's experiment.(NOT MCUNetV2 NAS)
exactly, Exactly, I want to reproduce the mobilenetV2 r144 w0.5 model shown in figure 6 of your paper(MCUNetV2,figure 6).
I generated Mbv2 model by tensorflow library, but it is too big to use in my board(F746gz-disc board)
I know that paper'model be used QAT, but I need other information for model generation.
Here is my model's inforamition. After learning fp32 MBV2 for only 1 epoch on the fp32 Imagenet-1000 dataset,I used post training quantization to quantize the model with int8 weights and activations. (but It was 2.2MB, not under 1MB.)
Could you please tell me what I need to do to make this model less than 1 MB like the MCUNetV2 paper?
*board information
*my model information
and I uploaded my model tfml file and tensorflow code.
more
import os
import tensorflow as tf
import config as c
from tqdm import tqdm
from tensorflow.keras import optimizers
from utils.data_utils import train_iterator
from utils.eval_utils import cross_entropy_batch, correct_num_batch, l2_loss
from tensorflow.keras.applications.mobilenet_v2 import MobileNetV2
from model.ResNet import ResNet
from model.ResNet_v2 import ResNet_v2
from test import test
import numpy as np
import os
import PIL
import PIL.Image
import tensorflow as tf
import pathlib
#Save the entire model as a SavedModel.
#!mkdir -p saved_model
model.save('saved_model/my_model')
def representative_data_gen():
for input_value in tf.data.Dataset.from_tensor_slices(train_ds).batch(1).take(100):
# Model has only one input so each data point has one element.
yield [input_value]
def representative_dataset():
for _ in range(100):
data = np.random.rand(1, 144, 144, 3)
yield [data.astype(np.float32)]
#PTQ part
#Convert the model to TensorFlow Lite format
converter = tf.lite.TFLiteConverter.from_saved_model('saved_model/my_model')
Hello, Thanks for your work.
I want to know how to make baseline model of MCUNetV2 paper's experiment.(NOT MCUNetV2 NAS)
exactly, Exactly, I want to reproduce the mobilenetV2 r144 w0.5 model shown in figure 6 of your paper(MCUNetV2,figure 6).
I generated Mbv2 model by tensorflow library, but it is too big to use in my board(F746gz-disc board)
I know that paper'model be used QAT, but I need other information for model generation.
Here is my model's inforamition. After learning fp32 MBV2 for only 1 epoch on the fp32 Imagenet-1000 dataset,I used post training quantization to quantize the model with int8 weights and activations. (but It was 2.2MB, not under 1MB.)
Could you please tell me what I need to do to make this model less than 1 MB like the MCUNetV2 paper?
*board information
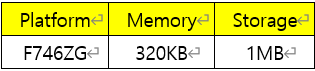
*my model information
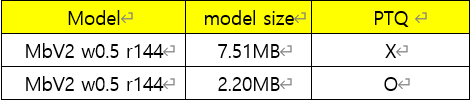
and I uploaded my model tfml file and tensorflow code.
*tfml model file
mobilenet_v2_quantized.zip
more
import os import tensorflow as tf import config as c from tqdm import tqdm from tensorflow.keras import optimizers from utils.data_utils import train_iterator from utils.eval_utils import cross_entropy_batch, correct_num_batch, l2_loss from tensorflow.keras.applications.mobilenet_v2 import MobileNetV2from model.ResNet import ResNet
from model.ResNet_v2 import ResNet_v2
from test import test
import numpy as np
import os
import PIL
import PIL.Image
import tensorflow as tf
import pathlib
os.environ['CUDA_VISIBLE_DEVICES'] = '0'
os.environ['CUDA_VISIBLE_DEVICES'] = '1'
checkpoint_path = "training_1/cp.ckpt"
checkpoint_dir = os.path.dirname(checkpoint_path)
data_dir = pathlib.Path("/home/poweroverwhelming/modelgen/ImageNet_ResNet_Tensorflow2.0/imagenet10/train")
image_count = len(list(data_dir.glob('/.JPEG')))
#training param
batch_size = 32
img_height = 144
img_width = 144
num_classes =1000
#directroy making
train_ds = tf.keras.utils.image_dataset_from_directory(
data_dir,
validation_split=0.2,
subset="training",
seed=123,
image_size=(img_height, img_width),
batch_size=batch_size)
val_ds = tf.keras.utils.image_dataset_from_directory(
data_dir,
validation_split=0.2,
subset="validation",
seed=123,
image_size=(img_height, img_width),
batch_size=batch_size)
#print class name
#nomalization
normalization_layer = tf.keras.layers.Rescaling(1./255)
normalized_ds = train_ds.map(lambda x, y: (normalization_layer(x), y))
image_batch, labels_batch = next(iter(normalized_ds))
first_image = image_batch[0]
#Notice the pixel values are now in
[0,1]
.#print(np.min(first_image), np.max(first_image))
AUTOTUNE = tf.data.AUTOTUNE
train_ds = train_ds.cache().prefetch(buffer_size=AUTOTUNE)
val_ds = val_ds.cache().prefetch(buffer_size=AUTOTUNE)
#training
model = MobileNetV2(input_shape=(144, 144, 3), alpha=0.5, weights=None, classes=1000)
model.compile(
optimizer='adam',
loss=tf.keras.losses.SparseCategoricalCrossentropy(from_logits=True),
metrics=['accuracy'])
cp_callback = tf.keras.callbacks.ModelCheckpoint(filepath=checkpoint_path,
save_weights_only=True,
verbose=1)
model.fit(train_ds,
validation_data=val_ds,
epochs=50)
#loss, acc = model.evaluate(test_images, test_labels, verbose=2)
#print("Untrained model, accuracy: {:5.2f}%".format(100 * acc))
#Save the entire model as a SavedModel.
#!mkdir -p saved_model
model.save('saved_model/my_model')
def representative_data_gen():
for input_value in tf.data.Dataset.from_tensor_slices(train_ds).batch(1).take(100):
# Model has only one input so each data point has one element.
yield [input_value]
def representative_dataset():
for _ in range(100):
data = np.random.rand(1, 144, 144, 3)
yield [data.astype(np.float32)]
#PTQ part
#Convert the model to TensorFlow Lite format
converter = tf.lite.TFLiteConverter.from_saved_model('saved_model/my_model')
#Perform post-training quantization
converter.optimizations = [tf.lite.Optimize.DEFAULT]
converter.target_spec.supported_ops = [tf.lite.OpsSet.TFLITE_BUILTINS_INT8]
converter.inference_input_type = tf.int8
converter.inference_output_type = tf.int8
converter.representative_dataset = representative_dataset
#Save the quantized model
tflite_model = converter.convert()
open("mobilenet_v2_imgnet10_r144_w05_quantizaed.tflite", "wb").write(tflite_model)
once again, Thank you for your work.
The text was updated successfully, but these errors were encountered: