You signed in with another tab or window. Reload to refresh your session.You signed out in another tab or window. Reload to refresh your session.You switched accounts on another tab or window. Reload to refresh your session.Dismiss alert
Thanks for sharing the code and dataset. The encoder-only architecture makes DDCP faster and lighter than other methods, I really like the idea. I try to reimplement the paper, however, some training details are missing in the paper.
loss
What are α and β used for the pre-train model? In the utilsV4.py it's all equal 1
Experiments
What are the total epochs for the pre-train model? In train.py, the default epochs=300
The text was updated successfully, but these errors were encountered:
Thanks for sharing the code and dataset. The encoder-only architecture makes DDCP faster and lighter than other methods, I really like the idea. I try to reimplement the paper, however, some training details are missing in the paper.
loss
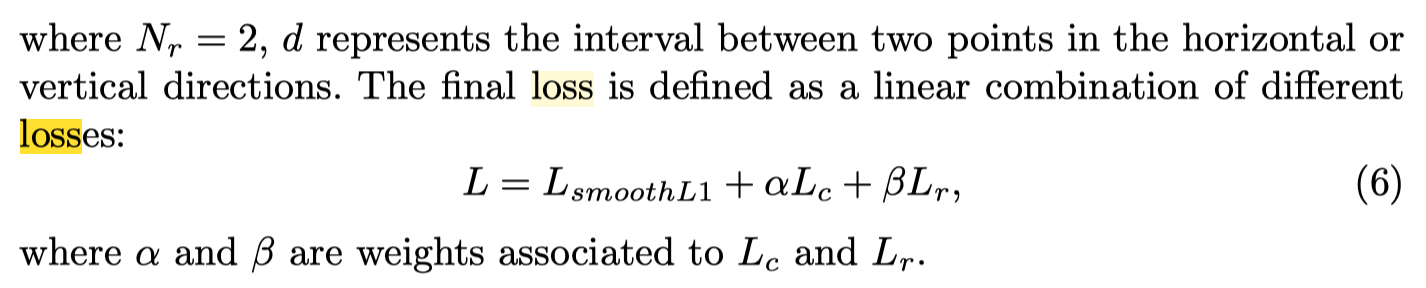
α
andβ
used for the pre-train model? In the utilsV4.py it's all equal1
Experiments

epochs=300
The text was updated successfully, but these errors were encountered: